How DeepSeek stacks up against popular AI models, in three charts
Category: News & Politics
Via: perrie-halpern • 4 weeks ago • 4 commentsBy: Jasmine Cui and Angela Yang
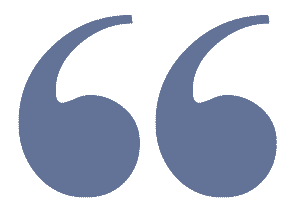
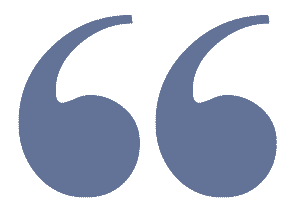
By Jasmine Cui and Angela Yang
DeepSeek, until recently a little-known Chinese artificial intelligence company, has made itself the talk of the tech industry after it rolled out a series of large language models that outshone many of the world's top AI developers.
DeepSeek released its buzziest large language model, R1, on Jan. 20. The AI assistant hit No. 1 on the Apple App Store in recent days, bumping OpenAI's long-dominant ChatGPT down to No. 2.
Its sudden dominance — and its ability to outperform top U.S. models across a variety of benchmarks — have both sent Silicon Valley into a frenzy, especially as the Chinese company touts that its model was developed at a fraction of the cost.
The shock within U.S. tech circles has ignited a reckoning in the industry, showing that perhaps AI developers don't need exorbitant amounts of money and resources in order to improve their models. Instead, researchers are realizing, it may be possible to make these processes efficient, both in terms of cost and energy consumption, without compromising ability.
R1 came on the heels of its previous model V3, which launched in late December. But Monday, DeepSeek released yet another high-performing AI model, Janus-Pro-7B, which is multimodal in that it can process various types of media.
Here are some features that make DeepSeek's large language models appear so unique.
Size
Despite being developed by a smaller team with drastically less funding than the top American tech giants, DeepSeek is punching above its weight with a large, powerful model that runs just as well on fewer resources.
That's because the AI assistant relies on a "mixture-of-experts" system to divide its large model into numerous small submodels, or "experts,"with each one specializing in handling a specific type of task or data. In contrast to the traditional approach, which uses every part of the model for every input, each submodel is activated only when its particular knowledge is relevant.
So even though V3 has a total of 671 billion parameters, or settings inside the AI model that it adjusts as it learns, it is actually only using 37 billion at a time, according to a technical report its developers published.
The company also developed a unique load-bearing strategy to ensure that no one expert is being overloaded or underloaded with work, by using more dynamic adjustments rather than a traditional penalty-based approach that can lead to worsened performance.
All these enable DeepSeek to employ a robust team of "experts" and to keep adding more, without slowing down the whole model.
It also uses a technique called inference-time compute scaling, which allows the model to adjust its computational effort up or down depending on the task at hand, rather than always running at full power. A straightforward question, for example, might only require a few metaphorical gears to turn, whereas asking for a more complex analysis might make use of the full model.
Together, these techniques make it easier to use such a large model in a much more efficient way than before.
Training cost
DeepSeek's design also makes its models cheaper and faster to train than those of its competitors.
Even as leading tech companies in the United States continue to spend billions of dollars a year on AI, DeepSeek claims that V3 — which served as a foundation for the development of R1 — took less than $6 million and only two months to build. And due to U.S. export restrictions that limited access to the best AI computing chips, namely Nvidia's H100s, DeepSeek was forced to build its models with Nvidia's less-powerful H800s.
One of the company's biggest breakthroughs is its development of a "mixed precision" framework, which uses a combination of full-precision 32-bit floating point numbers (FP32) and low-precision 8-bit numbers (FP8). The latter uses up less memory and is faster to process, but can also be less accurate.
Rather than relying only on one or the other, DeepSeek saves memory, time and money by using FP8 for most calculations, and switching to FP32 for a few key operations in which accuracy is paramount.
Some in the field have noted that the limited resources are perhaps what forced DeepSeek to innovate, paving a path that potentially proves AI developers could be doing more with less.
Performance
Despite its relatively modest means, DeepSeek's scores on benchmarks keep pace with the latest cutting-edge models from top AI developers in the United States.
R1 is nearly neck and neck with OpenAI's o1 model in the artificial analysis quality index, an independent AI analysis ranking. R1 is already beating a range of other models including Google's Gemini 2.0 Flash, Anthropic's Claude 3.5 Sonnet, Meta's Llama 3.3-70B and OpenAI's GPT-4o.
One of its core features is its ability to explain its thinking through chain-of-thought reasoning, which is intended to break complex tasks into smaller steps. This method enables the model to backtrack and revise earlier steps — mimicking human thinking — while allowing users to also follow its rationale.
V3 was also performing on par with Claude 3.5 Sonnet upon its release last month. The model, which preceded R1, had outscored GPT-4o, Llama 3.3-70B and Alibaba's Qwen2.5-72B, China's previous leading AI model.
Meanwhile, DeepSeek claims its newest Janus-Pro-7B surpassed OpenAI's DALL-E and Stable Diffusion's 3 Medium in multiple benchmarks.
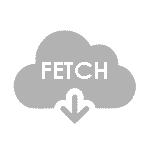
The key breakthrough here is that DeepSeek has apparently pioneered an algorithm that is dramatically more efficient in terms of the test-time (thinking) computations. This makes it possible to produce applications like ChatGPT with far less computation (and actually enable them to run on private hardware rather than on server farms).
Since this is open-source, the entire planet can compile and run their code. This can all be absolutely verified by third parties. Plus the research paper has been released. This is a gift to the planet.
Not expected, but here it is.
Yes!
Seems to me the innovation of DeepSeek has been to combine parallel computing with distributed computing. What has been described in the pop press sounds very much like how a team would work -- a 'power of the crowd' approach. In crude, imprecise terms, it's the difference between one super intelligent brain and a group of moderately intelligent brains. (Is this a case of diversity winning out?)
No wonder investors are pissed. I wasn't aware that DeepSeek was open source (meaning it can't be bought and buried). Just throwing money at AI won't provide dominance or monopoly control now. Has the hegemony of the Microsoft business model doomed the US efforts to dominate AI? It's certainly not looking good for Copilot, at the least.
It is mostly an efficient algorithm that is highly effective at avoiding doing work that is least likely to give strong results and focusing on that which most likely will.
This is a common principle of computer science that has been applied to the problem of making efficient test-time computation against LLMs.
Well, yes, my description was imprecise. DeepSeek utilizes a distributed Mixture of Experts architecture that essentially distributes query processing across specially trained neural subnetworks. In oversimplified terms DeepSeek is spreading the processing tasks across a group of submodels instead of utilizing one massive model. The DeepSeek LLM has been subdivided into 'expert' sub networks that are only active when needed. As the seeded article suggests that increases speed and efficiency but can adversely affect accuracy. IMO the accuracy of the 'expert' subnets is more likely due to inadequacies in training rather than limitations of the architecture.
My understanding is that ChatGPT distributes query processing and response across more processors. Again oversimplified, that's basically just a brute force approach of running the LLM on a bigger computer.