New hardware offers faster computation for artificial intelligence, with much less energy
Category: Health, Science & Technology
Via: hallux • 3 years ago • 4 commentsBy: Massachusetts Institute of Technology
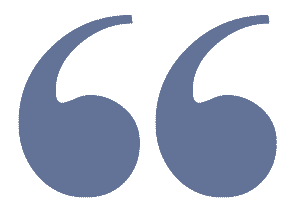
As scientists push the boundaries of machine learning, the amount of time, energy, and money required to train increasingly complex neural network models is skyrocketing. A new area of artificial intelligence called analog deep learning promises faster computation with a fraction of the energy usage.
Programmable resistors are the key building blocks in analog deep learning, just like transistors are the core elements for digital processors. By repeating arrays of programmable resistors in complex layers, researchers can create a network of analog artificial "neurons" and "synapses" that execute computations just like a digital neural network. This network can then be trained to achieve complex AI tasks like image recognition and natural language processing.
A multidisciplinary team of MIT researchers set out to push the speed limits of a type of human-made analog synapse that they had previously developed. They utilized a practical inorganic material in the fabrication process that enables their devices to run 1 million times faster than previous versions, which is also about 1 million times faster than the synapses in the human brain .
Moreover, this inorganic material also makes the resistor extremely energy-efficient. Unlike materials used in the earlier version of their device, the new material is compatible with silicon fabrication techniques. This change has enabled fabricating devices at the nanometer scale and could pave the way for integration into commercial computing hardware for deep-learning applications.
"With that key insight, and the very powerful nanofabrication techniques we have at MIT.nano, we have been able to put these pieces together and demonstrate that these devices are intrinsically very fast and operate with reasonable voltages," says senior author Jesús A. del Alamo, the Donner Professor in MIT's Department of Electrical Engineering and Computer Science (EECS). "This work has really put these devices at a point where they now look really promising for future applications."
"The working mechanism of the device is electrochemical insertion of the smallest ion, the proton , into an insulating oxide to modulate its electronic conductivity. Because we are working with very thin devices, we could accelerate the motion of this ion by using a strong electric field, and push these ionic devices to the nanosecond operation regime," explains senior author Bilge Yildiz, the Breene M. Kerr Professor in the departments of Nuclear Science and Engineering and Materials Science and Engineering.
"The action potential in biological cells rises and falls with a timescale of milliseconds, since the voltage difference of about 0.1 volt is constrained by the stability of water," says senior author Ju Li, the Battelle Energy Alliance Professor of Nuclear Science and Engineering and professor of materials science and engineering, "Here we apply up to 10 volts across a special solid glass film of nanoscale thickness that conducts protons, without permanently damaging it. And the stronger the field, the faster the ionic devices."
These programmable resistors vastly increase the speed at which a neural network is trained, while drastically reducing the cost and energy to perform that training. This could help scientists develop deep learning models much more quickly, which could then be applied in uses like self-driving cars, fraud detection, or medical image analysis.
"Once you have an analog processor, you will no longer be training networks everyone else is working on. You will be training networks with unprecedented complexities that no one else can afford to, and therefore vastly outperform them all. In other words, this is not a faster car, this is a spacecraft," adds lead author and MIT postdoc Murat Onen.
The research is published today in Science .
Accelerating deep learning
Analog deep learning is faster and more energy-efficient than its digital counterpart for two main reasons. "First, computation is performed in memory, so enormous loads of data are not transferred back and forth from memory to a processor." Analog processors also conduct operations in parallel. If the matrix size expands, an analog processor doesn't need more time to complete new operations because all computation occurs simultaneously.
The key element of MIT's new analog processor technology is known as a protonic programmable resistor. These resistors, which are measured in nanometers (one nanometer is one billionth of a meter), are arranged in an array, like a chess board.
In the human brain, learning happens due to the strengthening and weakening of connections between neurons, called synapses. Deep neural networks have long adopted this strategy, where the network weights are programmed through training algorithms. In the case of this new processor, increasing and decreasing the electrical conductance of protonic resistors enables analog machine learning.
The conductance is controlled by the movement of protons. To increase the conductance, more protons are pushed into a channel in the resistor, while to decrease conductance protons are taken out. This is accomplished using an electrolyte (similar to that of a battery) that conducts protons but blocks electrons.
To develop a super-fast and highly energy efficient programmable protonic resistor, the researchers looked to different materials for the electrolyte. While other devices used organic compounds, Onen focused on inorganic phosphosilicate glass (PSG).
PSG is basically silicon dioxide, which is the powdery desiccant material found in tiny bags that come in the box with new furniture to remove moisture. It is also the most well-known oxide used in silicon processing. To make PSG, a tiny bit of phosphorus is added to the silicon to give it special characteristics for proton conduction.
Onen hypothesized that an optimized PSG could have a high proton conductivity at room temperature without the need for water, which would make it an ideal solid electrolyte for this application. He was right.
Surprising speed
PSG enables ultrafast proton movement because it contains a multitude of nanometer-sized pores whose surfaces provide paths for proton diffusion. It can also withstand very strong, pulsed electric fields. This is critical, Onen explains, because applying more voltage to the device enables protons to move at blinding speeds.
"The speed certainly was surprising. Normally, we would not apply such extreme fields across devices, in order to not turn them into ash. But instead, protons ended up shuttling at immense speeds across the device stack, specifically a million times faster compared to what we had before. And this movement doesn't damage anything, thanks to the small size and low mass of protons. It is almost like teleporting," he says.
"The nanosecond timescale means we are close to the ballistic or even quantum tunneling regime for the proton, under such an extreme field," adds Li.
Because the protons don't damage the material, the resistor can run for millions of cycles without breaking down. This new electrolyte enabled a programmable protonic resistor that is a million times faster than their previous device and can operate effectively at room temperature , which is important for incorporating it into computing hardware.
Thanks to the insulating properties of PSG, almost no electric current passes through the material as protons move. This makes the device extremely energy efficient, Onen adds.
Now that they have demonstrated the effectiveness of these programmable resistors, the researchers plan to reengineer them for high-volume manufacturing, says del Alamo. Then they can study the properties of resistor arrays and scale them up so they can be embedded into systems.
At the same time, they plan to study the materials to remove bottlenecks that limit the voltage that is required to efficiently transfer the protons to, through, and from the electrolyte.
"Another exciting direction that these ionic devices can enable is energy efficient hardware to emulate the neural circuits and synaptic plasticity rules that are deduced in neuroscience, beyond analog deep neural networks," adds Yildiz.
"The collaboration that we have is going to be essential to innovate in the future. The path forward is still going to be very challenging, but at the same time it is very exciting," del Alamo says.
Co-authors include Frances M. Ross, the Ellen Swallow Richards Professor in the Department of Materials Science and Engineering; postdocs Nicolas Emond and Baoming Wang; and Difei Zhang, an EECS graduate student.
The joke will be on us if both A-I and God decide not to communicate with us and only with 'themselves'.
I'm sure they will find a better source of power than using pod people as batteries.
Keep your soul pure, the 'gods' find them tastier and they do love a banquet.
This makes great sense. The core of AI neural networks is the neuron which will accept an arbitrary number of continuous values (high precision decimal but often between 0 and 1) and produce (if an action potential threshold is met) continuous values which serve as input to an arbitrary number of neurons in the next layer (typically).
The computation is predominantly a linear algebra algorithm (matrix operations). Actually two: one to process input and produce the 'answer' of a neural network and another to backtrack to fine tune the neural network to reduce error (and thus, to learn). To gain speed with today's computers AI algorithms often tap graphics processors (which are designed to perform matrix operations). So if we had hardware that intrinsically delivered continuous (analog) values and could perform matrix operations like game video cards do, it would certainly speed up AI applications (at least those using neural networks).
Very cool.
By the way, this technology need not be ubiquitous. It can serve to train a neural network (to cause it to learn) but then when the training is done, the trained values (the weights assigned to links between neurons) can be transferred to a neural network using standard computing hardware. And, of course, the neural network (on its special hardware) can also simply reside in the cloud and be accessed dynamically by ordinary devices.
So the extremely expensive, time consuming process of training (learning) can be done on hardware designed for the task but operation can be on normal devices.