4 Predictions About The Wild New World Of Text-To-Image AI
Category: Photography & Art
Via: hal-a-lujah • 2 years ago • 14 commentsBy: Rob Toews (Forbes)
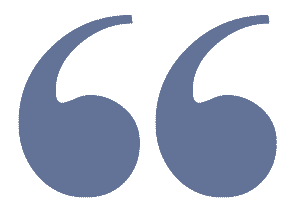
Give it a try.
https://beta.dreamstudio.ai/dream
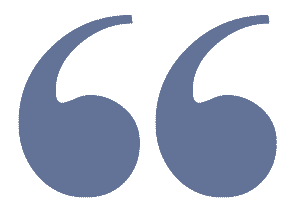
A powerful new form of artificial intelligence has burst onto the scene and captured the public's imagination in recent months: text-to-image AI.
Text-to-image AI models generate original images based solely on simple written inputs. Users can input any text prompt they like—say, "a cute corgi lives in a house made out of sushi"—and, as if by magic, the AI will produce a corresponding image. (See above for this example; scroll down for some more.)
These models produce images that have never existed in the world nor in anyone's imagination. They are not simple manipulations of existing images on the Internet; they are novel creations, breathtaking in their originality and sophistication.
The most well-known text-to-image model is OpenAI's DALL-E. OpenAI debuted the original DALL-E model in January 2021. DALL-E 2, its successor, was announced in April 2022. DALL-E 2 has attracted widespread public attention, catapulting text-to-image technology into the mainstream.
In the wake of the excitement around DALL-E 2, it hasn't taken long for competitors to emerge. Within weeks, a lightweight open-source version dubbed "DALL-E Mini" went viral. Unaffiliated with OpenAI or DALL-E, DALL-E Mini has since been rebranded as Craiyon following pressure from OpenAI.
In May, Google published its own text-to-image model, named Imagen. (All the images included in this article come from Imagen.)
Soon thereafter, a startup named Midjourney emerged with a powerful text-to-image model that it has made available for public use. Midjourney has seen astonishing user growth: launched only two months ago, the service has over 1.8 million users in its Discord group as of this writing. Midjourney has recently been featured on the cover of The Economist and on John Oliver's late-night TV show.
Another key entrant in this category is Stability.ai, the startup behind the Stable Diffusion model. Unlike any other competitor, Stability.ai has publicly released all the details of its AI model, publishing the model's weights online for anyone to access and use. This means that, unlike DALL-E or Midjourney, there are no filters or limitations on what Stable Diffusion can be used to generate—including violent, pornographic, racist, or otherwise harmful content.
Stability.ai's completely unrestricted release strategy has been controversial. On the other hand, the company's unapologetically open ethos is helping it build a strong community of developers and users around its platform, which may prove to be a valuable competitive advantage.
There is much to be said about the groundbreaking technology that underlies today's generative AI, but one key innovation in particular is worth briefly highlighting: diffusion models. Originally inspired by concepts from thermodynamics, diffusion models have seen a surge of popularity over the past year, rapidly displacing generative artificial networks (GANs) as the go-to method for AI-based image generation. DALL-E 2, Imagen, Midjourney and Stable Diffusion all use diffusion models.
In a nutshell, diffusion models learn by corrupting their training data with incrementally added noise and then figuring out how to reverse this noising process to recover the original image. Once trained, diffusion models can then apply these denoising methods to synthesize novel "clean" data from random input.
Stepping back, what are we to make of all the recent activity and buzz in this space? Where will things go from here? Below are four predictions that aim to cut through the noise and give you original perspectives on the wild new world of generative AI.
(Before reading any further: take a few moments to experiment with these AI models for yourself if you haven't already. There is no substitute for trying them out first-hand. They are free of charge and dead simple to use, requiring nothing but a quick sign-up. Go here to try Stable Diffusion and go here to try Midjourney.)
1. A lot of venture capital is going to flow into this category over the next twelve months.
A narrative has begun to percolate through the world of venture capital that text-to-image AI is "the next big thing." There is no question that the technology is extraordinary; time will tell whether and how it will serve as the foundation for massive, enduring businesses.
Regardless, expect a flurry of venture investment in the space in the near term as investors seek to ride this wave.
The opening salvo here came last week, with reports that Stability.ai is raising a whopping $100 million at a valuation of up to $1 billion from blue-chip investors like Lightspeed and Coatue.
This will not be the last mega-deal in this category. Midjourney, for example, is likely fielding a flood of inbound investor interest at the moment. Midjourney has been self-funded to this point by founder David Holz (former Leap Motion CTO/cofounder)—but don't be surprised if the company soon decides to fill its coffers with venture capital dollars in order to compete and scale in this increasingly fast-moving ecosystem.
Many new text-to-image startups will emerge in the months ahead, with different visions and approaches to commercializing this powerful new technology. Even in today's adverse market conditions, venture capitalists will eagerly fund many of them. Which leads us to our next point.....
2. The biggest business opportunities and best business models for this technology have yet to be discovered.
The primary use case that has driven adoption of text-to-image AI to date has been sheer novelty and curiosity on the part of individual users. And no wonder—as anyone who has played around with one of these models can attest, it is an exhilarating and engaging experience, especially at first.
But over the longer term, casual use by individual hobbyists is not by itself likely to sustain massive new businesses.
What use cases will unleash vast enterprise value creation and present the most compelling business opportunities for this technology? Put simply, what are the "killer apps" for text-to-image AI?
One application that immediately comes to mind is advertising. Advertising is visual in nature, making it a natural fit for these generative AI models. And after all, advertising powers the business models of technology giants like Alphabet and Facebook, among the most successful businesses in history.
Some brands, for instance Kraft Heinz, have already begun experimenting with AI models like DALL-E 2 to produce new advertising content. No doubt we will see a lot more of this. But—to be frank—let us all hope that we find more meaningful use cases for this incredible new technology than simply more advertising.
Taking a step back, consider that these AI models make it possible to generate and iterate upon any visual content quickly, affordably, and imaginatively, without the need for any special expertise or training. When we frame the scope of the technology this broadly, it becomes more evident that all sorts of transformative, disruptive business opportunities should emerge.
Perhaps the most intuitive use case for this technology is to create art. The global market size for fine art is $65 billion. Even setting aside this high end of the market, there are numerous more quotidian uses for art to which text-to-image AI could be profitably applied: book covers, magazine covers, postcards, posters, music album designs, wallpaper, digital media, and so on.
Take stock images as an example. Stock imagery may seem like a relatively niche market, but by itself it represents a multi-billion-dollar opportunity, with publicly traded competitors including Getty Images and Shutterstock. These businesses face existential disruption from generative AI.
Longer term, the design (and thus production) of any physical product—cars, furniture, clothes—could be transformed as generative AI models are used to dream up novel features and designs that captive consumers.
Relatedly, text-to-image AI may influence architecture and building design by "proposing" unique, unexpected new structures and layouts that in turn inspire human architects. Initial work along these lines is already being pursued today.
Prompt: "A small cactus wearing a straw hat and[+][-] neon sunglasses in the Sahara desert." Courtesy of Google Brain's Imagen model.Source: Google
Alongside the question of killer applications is the related but distinct topic of how the competitive landscape in this category will evolve, and in turn which product and go-to-market strategies will prove most effective.
Early movers like OpenAI and Midjourney have positioned themselves as horizontal, sector-agnostic providers of the core AI technology. They have built general-purpose text-to-image models, made them available to customers via API (with pricing on a pay-per-use basis), and left it to users to discover their own use cases.
Will one or more horizontal players achieve massive scale by offering a foundational text-to-image platform on top of which an entire ecosystem of diverse applications is built? If so, will it be winner-take-all? As the technology eventually becomes commoditized, what would the long-term moats for such a business be?
Or as the sector matures and different use cases come into focus, will there be more value in building purpose-built, specialized solutions for particular applications?
One could imagine, say, a text-to-image solution built specifically for the auto industry for the design of new vehicle models. In addition to the AI model itself being fine-tuned on training data for this particular use case, such a solution might include a full SaaS product suite and a well-developed user interface built to integrate seamlessly into car designers' overall workflows.
Another key strategic issue concerns the core AI models themselves. Can these models serve as a sustainable source of defensibility for companies, or will they quickly become commoditized? Recall that Stable Diffusion, one of today's leading text-to-image models, has already been fully open-sourced, with all of its weights freely available online. How often and under what conditions will it make sense for a new startup to train its own proprietary text-to-image models internally, as opposed to leveraging what has already been built from the open-source community or from another company?
We cannot yet know the answer to any of these questions with certainty. The only thing we can be sure of is that this field will develop in surprising, unexpected ways in the months and years ahead. Part of the magic of new technology is that it unlocks previously unimaginable possibilities. When dial-up internet first became available, who predicted YouTube? When the first smartphones debuted, who saw Uber coming?
It is entrepreneurs who will ultimately answer these questions by envisioning and building the future themselves.
3. Text-to-image AI will unleash a hornet's nest of copyright, legal, and ethical issues. Don't expect these to slow the technology down.
Any new technology that offers to profoundly shake up the status quo will generate frictions and challenges with existing societal norms and policy frameworks. Generative AI is no exception.
There are a number of big-picture issues that this technology raises: the ever-present topic of AI-driven job displacement, the looming threat of deepfakes that these models intensify, the philosophical question of what constitutes true art and whether AI can ever create it. There are no easy answers to these questions, and the public discourse about them will continue for years.
There is one near-term issue that is worth briefly touching on here: the question of who owns and has the right to commercialize the images that these models produce.
Can the person who came up with a text prompt and fed it into an AI model take the resulting image and do whatever he or she likes with it (including in a commercial setting)? Or does the organization that built the AI model retain rights to all media that the model produces? What if the AI model is open source?
Complicating things further, consider the fact that the way companies like Google and OpenAI create these models in the first place is by training them on vast troves of publicly available images that those companies do not own, including the work of countless other artists, designers and organizations.
These questions are not just theoretical; they will have very real and immediate business consequences. Whether and how these issues are resolved will have a significant impact on the strategies and opportunities available to companies working with this technology. Entrepreneurs and investors need to pay attention.
"If DALL-E is adopted in the way I think [OpenAI] envisions it, there's going to be a lot of revenue generated by the use of the tool," said Bradford Newman, an AI-focused lawyer at law firm Baker & McKenzie. "And when you have a lot of players in the market and issues at stake, you have a high chance of litigation."
OpenAI's currently stated policy is that DALL-E's individual users get full rights to commercialize the images that they create with the model—including the right to reprint, sell, or merchandise the images—but that OpenAI retains ultimate ownership over the original images. Midjourney's terms of service say something similar.
But when high-stakes disputes involving these images inevitably get litigated, will courts see it this way? This is uncharted territory; no direct legal precedent exists.
Jim Flynn, senior partner at law firm Epstein Becker & Green, provided a concrete example that illustrates the dynamics at play: "If I were representing one of the advertising agencies, or the clients of the advertising agencies, I wouldn't advise them to use this software to create a campaign, because I do think the AI provider would [currently] have some claims to the intellectual property. I'd be looking to negotiate something more definitive."
Ultimately, these issues should be seen not as showstoppers for the technology but rather as unresolved points that will be in play as this nascent industry barrels ahead at full speed. Make no mistake: legal ambiguity will not deter entrepreneurs and technologists from pushing forward the state of the art in this field and from building businesses that bring this technology to the masses.
An OpenAI spokesperson summed it up well: "Copyright law has adapted to new technology in the past and will need to do the same with AI-generated content."
Prompt: "Teddy bear swimming at the Olympics 400m[+][-] Butterfly event." Courtesy of Google Brain's Imagen model.Source: Google
4. This technology is going to get much more mind-blowing—quickly.
As impressive as today's text-to-image models are, we are still in the earliest innings of the proliferation of generative AI. Text-to-image is just the beginning.
The most natural next step will be text-to-video AI models: generative models that can take in a text description and produce not just a static image but a video of specified length.
Needless to say, text-to-video is a significantly more complex technical challenge than text-to-image. For one thing, it requires vastly greater computing resources; for another, well-annotated video training data is scarce.
But the opportunity here is tremendous. From TikTok to Netflix, video has become the dominant medium for our digital lives. According to Cisco, over 80% of all data on the internet today is video. The ability to easily and cheaply generate new video content on demand will be transformative, from entertainment to social media to marketing and beyond.
The most promising academic research on this topic is CogVideo, a large-scale text-to-video model published in May 2022. Just two days ago, video AI startup Runway announced the upcoming release of text-to-video tools on its platform, which it said are "coming soon." Runway appears to be collaborating with Stability.ai on this effort.
Another avenue for future innovation will be AI models that generate 3-D digital content (as opposed to the 2-D outputs from models like DALL-E). This technology will have enormous implications for areas including gaming, animated filmmaking and the metaverse.
One final tantalizing possibility: imagine pairing a generative AI model with a 3-D printer to enable text-to-real-world-object-generation. As one Twitter user colorfully described it: "literally conjuring objects from incantations."
To be sure, this remains out of reach today. But the core technology building blocks to make something like this a reality are basically in place.
The future is going to be mind-blowing—and it's going to be here sooner than you think.
Follow me on Twitter. Rob ToewsEditorial StandardsCorrectionsReprints & Permissions
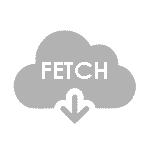
This will blow your mind. It’s free - check the link at the top of the page. You just input random words and it generates an image in about ten seconds.
Example: corn + salamander
Very cool, thanks.
Clown + Pope
You can really confuse it by entering things that can’t exist in reality.
Fish riding bicycle
I'm only able to generate art from my photos that I have on my hard drive. The above image is from this photo
That’s weird. I’m using my phone and it’s generating crazy shit.
Trump Frankenstein
I like that one
I’ve found that the text never makes sense. I’m sure that will improve with time.
Another reason to die sooner than later ...
The day words are lost in the malaise is the day we are lost in the daze.
Sorry but not a fan of a bunch of fake images being made. Real photography will be sidelined by fake.
Then who is to say if something is real or not.
Next thing they will have is be able to copy people and out them in scenarios that they never were.
I don’t find the two to be remotely similar. It’s real vs. surreal. May as well get used to it … and consider it in your future stock portfolio.
Curmudgeon + surreal